Today’s News Glance
🛒 ChatGPT Shopping Experience Upgrade: AI Assistant Turning into a Smart Shopping Guide?
🚀 Alibaba Qwen3 Large Model Fully Open Sourced: Performance Leap, Reshaping the Open Source Landscape
💡 Tsinghua et al. Propose DeepNote: New Breakthrough in RAG Technology, Exploring New Depths of Knowledge Acquisition
☁️ Google AI Application Expansion & Photos Optimization: Enterprises Accelerate Implementation, User Experience Fine-tuning
01 🛒 ChatGPT Shopping Experience Upgrade: AI Assistant Turning into a Smart Shopping Guide?
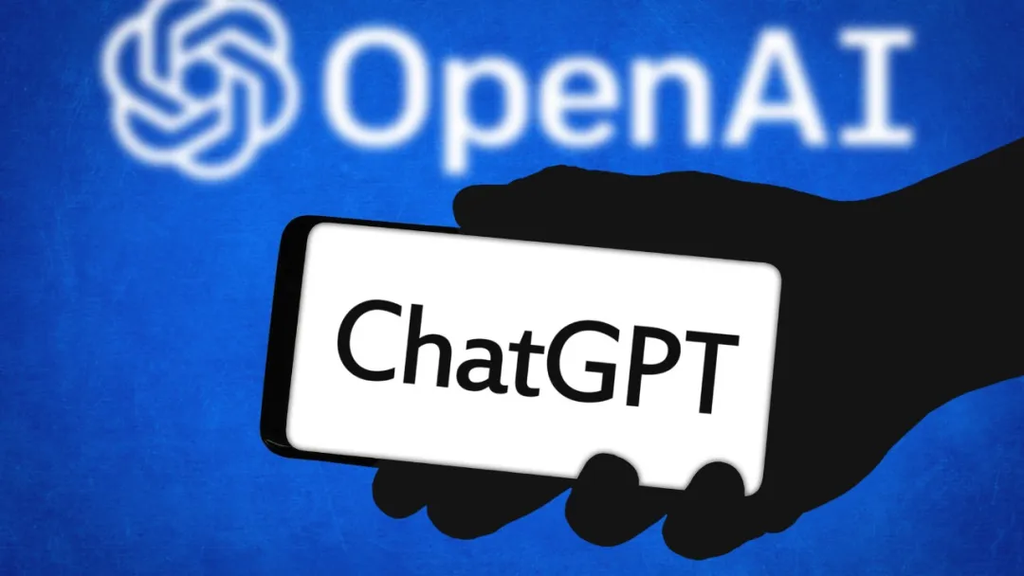
OpenAI recently launched integrated shopping features for ChatGPT, allowing users to directly search for products, compare prices and reviews within the chat, and jump to purchase via links. This update blurs the lines between AI assistants and search engines, marking ChatGPT’s shift from an information tool towards a transaction platform, aiming to provide a more natural, conversational shopping discovery experience.
Highlights
- Seamless Shopping: Users can complete the entire process of product discovery and comparison within the ChatGPT conversation, without needing to jump between multiple websites.
- Optimized Recommendations: Search results are presented as visual cards, including images, real-time prices, and user review summaries, aggregating key decision-making information.
- Convenient Purchase: Each recommended product comes with a direct purchase link; clicking it redirects to the retailer’s or brand’s official website, shortening the purchase path.
- Objective & Independent: OpenAI claims search results are not paid advertisements and are recommended based on the product’s relevance. However, collaboration with partners ensures information accuracy, and its impact needs monitoring.
- Full Rollout: The feature began rolling out gradually to all user tiers starting April 28th, indicating its functional positioning.
Value Insights
- For AI & E-commerce Professionals: This opens up a new entry point for product guidance, potentially changing user decision journeys and requiring adjustments to SEO/SEM strategies to adapt to AI recommendations. It also intensifies competition with services like Google Shopping in the product search market. Combined with the “memory” feature, the potential for personalization is huge, but it also brings challenges related to data privacy and algorithmic fairness.
- For General Users: It offers a more natural and convenient way to obtain shopping information, especially suitable for comparing multiple products. However, users should view the “non-advertisement” claim rationally and may need to weigh information depth against response speed (early tests suggest it might be slower than traditional search).
Recommended Reading
- Tom’s Guide: ChatGPT Shopping Update
- BGR: ChatGPT Search Upgrades for Shopping
- Search Engine Land: OpenAI Adds Shopping Features
02 🚀 Alibaba Qwen3 Large Model Fully Open Sourced: Performance Leap, Reshaping the Open Source Landscape

On April 28th, Alibaba officially released and fully open-sourced its latest generation large language model series, Qwen3. The series includes six dense models ranging from 0.6B to 32B parameters and two MoE (Mixture of Experts) models (up to 235B parameters), all under the Apache 2.0 license. Official benchmarks show Qwen3 demonstrates strong performance in tasks like coding, math, and multilingual capabilities, with the flagship model comparable to top industry levels. It’s now available for download on platforms like Hugging Face.
Highlights
- Fully Open Source: A total of 8 models (6 Dense + 2 MoE), from small to large, are released under the Apache 2.0 license, significantly lowering the barrier to entry.
- Leading Performance: The flagship MoE model’s performance rivals top closed-source models, the medium MoE surpasses previous large models, and smaller models also achieve a performance leap.
- Hybrid Inference: MoE models feature a unique “thinking/non-thinking mode” switching capability, balancing the depth needed for complex reasoning with the efficiency of fast responses.
- Open Access: Model weights are available on mainstream platforms like Hugging Face, ModelScope, and Kaggle, making them easily accessible to global developers.
- Ecosystem Support: Official recommendations include various mature cloud-based (e.g., vLLM) and local (e.g., Ollama, llama.cpp) deployment frameworks, reducing usage difficulty.
- Long Context: Most models natively support 32K or 128K context, with MoE models extendable to 131K, showing strong long-text processing capabilities.
- Multilingual Enhancement: Pre-training data covers 119+ languages, significantly improving multilingual processing abilities.
Value Insights
- For AI Developers and Researchers: Qwen3 offers powerful, diverse, and permissively licensed open-source models, especially the efficient MoE models, lowering the development threshold for high-performance AI applications. Good ecosystem support simplifies deployment, promising to accelerate technological iteration and innovation.
- For General Users: More powerful open-source models mean a future with more diverse, intelligent, and potentially more economical AI applications, enhancing user experience. Additionally, the open sourcing of technology aids knowledge dissemination.
Recommended Reading
03 💡 Tsinghua et al. Propose DeepNote: New Breakthrough in RAG Technology
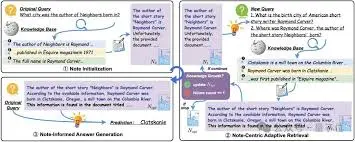
Addressing the pain points of existing RAG (Retrieval-Augmented Generation) technologies—namely insufficient or redundant information when tackling complex problems—a research team from Tsinghua University and other institutions has proposed the DeepNote framework. Its core innovation lies in introducing a “notes” mechanism as a dynamic medium for accumulating and refining knowledge. Through adaptive retrieval and note-based generation, it integrates external knowledge more deeply and effectively. Experiments demonstrate that DeepNote significantly outperforms baseline methods on complex question-answering tasks.
Highlights
- Notes as a Medium: Uses “notes” as a dynamic carrier for the model to understand, refine, and summarize acquired knowledge, rather than simply stacking information.
- Adaptive Retrieval: Intelligently decides whether and how to perform the next retrieval round based on assessing current knowledge gain, reducing blindness and noise.
- Deep Integration: Facilitates effective fusion and interaction of information across retrieval rounds by iteratively updating notes, generating answers using the optimal notes.
- Superior Performance: Comprehensively surpasses existing RAG methods on multiple QA datasets, with up to a 20.1% improvement over basic RAG.
- Addresses Pain Points: Specifically targets core RAG challenges in complex QA, such as knowledge insufficiency, retrieval redundancy, and inadequate integration.
Value Insights
- For AI Practitioners and Researchers: DeepNote provides a new paradigm for enhancing LLM reliability and the ability to process complex information, particularly suitable for knowledge-intensive applications. Its “knowledge construction” rather than simple “information retrieval” approach opens new research directions in the RAG field and may inspire the design of more intelligent AI Agents.
- For General Users: This implies that future AI applications answering complex questions could provide more accurate, reliable, and well-organized answers. AI will not only find information but also perform knowledge “digestion and integration.”
Recommended Reading
04 ☁️ Google AI Application Expansion & Photos Optimization: Enterprises Accelerate Implementation, User Experience Fine-tuning

Google Cloud disclosed that the number of enterprise Generative AI (GenAI) use cases on its platform surged from 101 to 601 in one year, an increase of nearly 500%. This indicates GenAI is moving from experimentation to large-scale deployment, widely adopted by industry giants like Uber and Citi. Simultaneously, addressing user feedback, Google added a feature in the Android version of Google Photos allowing users to double-tap the “Search” icon to quickly switch back to the classic search mode, optimizing the user experience for the sometimes slower responses of the AI search feature (Ask Photos).
Highlights
- Enterprise Acceleration: Real-world GenAI application cases on Google Cloud grew nearly 5-fold in a year, showing enterprises are accelerating AI rollout from pilot projects to production environments.
- Giant Adoption: Industry leaders (e.g., Uber, Citi, Benz, Samsung) are actively using Google Cloud AI (Vertex AI, Gemini) to optimize core business processes.
- Photos Optimization: Responding to feedback about the speed of “Ask Photos” AI search, Google added an option in Android Photos to conveniently switch back to classic search.
- Convenient Switching: Android users can double-tap the bottom “Search” icon to bypass AI search and directly enter the traditional search interface, improving operational efficiency.
Researcher’s Thoughts
- For AI and Related Industry Professionals: The surge in enterprise use cases is a strong signal of GenAI’s widely recognized business value, highlighting the importance of enterprise-grade AI platforms. Meanwhile, the Photos adjustment serves as a reminder to prioritize user experience; even advanced features must consider usability and user habits.
- For General Users: Widespread enterprise AI adoption signals that users can expect more intelligent and efficient services in the future. The Photos optimization offers a choice between new and old features, enhancing the application’s user-friendliness.
Recommended Reading
Today’s Summary
Today’s AI industry dynamics were rich and varied, showcasing parallel advancements at the application, model, and fundamental research layers. OpenAI extended ChatGPT’s capabilities into online shopping, blurring the lines between AI assistants and search engines, sparking thought about future e-commerce entry points and information objectivity. Alibaba’s full open-sourcing of the Qwen3 series, especially its high-performance MoE models and friendly license, provides powerful new tools for global developers, further fueling the open-source AI boom. On the fundamental research front, the DeepNote RAG framework proposed by Tsinghua University and others offers new approaches to solving LLM knowledge integration challenges in complex Q&A through its innovative “notes” mechanism. Concurrently, the explosive growth of Google Cloud AI applications on the enterprise side, coupled with its continuous optimization of AI feature user experience in consumer products, collectively demonstrates that AI technology is rapidly moving from frontier exploration to widespread real-world implementation.