Today’s News Highlights
📰 Tencent Hunyuan Custom’s Stunning Open Source: Ushering in a “Consistency” Revolution for Video Generation?
💻 OpenAI Connects to GitHub: ChatGPT Transforms into a “Code Whisperer” for Deep Codebase Interpretation
💡 Alibaba ZeroSearch Emerges: AI Training Bids Farewell to the “Money-Burning” Mode?
⚖️ US AI Chip Export Policy Shifts Direction: Adding New Variables to the Global AI Landscape
01 📰 Tencent Hunyuan Custom’s Stunning Open Source: Ushering in a “Consistency” Revolution for Video Generation?
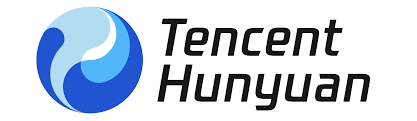
Tencent has recently stirred up the artificial intelligence field again, officially announcing the comprehensive open-sourcing of its latest multi-modal customized video generation large model – HunyuanCustom. This model, built upon the powerful Hunyuan video generation framework, not only supports various input methods such as text, images, audio, and video, but has also achieved significant breakthroughs in the industry’s highly关注 “subject consistency.” It is claimed that its performance surpasses existing open-source solutions like Pika and VACE on multiple benchmarks. This open-source initiative is considered thorough, encompassing model weights, inference code, and complete model algorithms, with a parameter count as high as 13 billion, making it the largest open-source model in the video generation field to date. This move not only signifies the full open-sourcing of Tencent’s Hunyuan series large models but also demonstrates Tencent’s commitment to promoting the prosperity and development of the entire AI video creation ecosystem.
Key Highlights
- Multi-modal Input: HunyuanCustom can flexibly integrate text descriptions, static images, audio clips, and even other video materials as input conditions to generate highly customized video content.
- Subject Consistency: It focuses on tackling the technical bottleneck of maintaining the coherent identity characteristics of the core subject (person or object) in continuous video frames during the video generation process.
- Excellent Performance: In single-subject and multi-subject scene evaluations, HunyuanCustom demonstrates excellent performance in ID consistency, video realism, and text-to-video alignment accuracy.
- Thorough Open Source: It has open-sourced model weights with up to 13 billion parameters, complete inference code, and core algorithms, aiming to significantly lower the application threshold for the industry.
- Broad Scenarios: With its powerful multi-modal processing and customized generation capabilities, it can handle various downstream application tasks such as virtual human advertising, online try-on, and singing avatars.
Value Insights
- For Practitioners: The open-sourcing of HunyuanCustom, especially its massive 13 billion parameter scale and technological breakthrough in subject consistency, provides developers in video content creation, digital human development, metaverse construction, and other fields with powerful tools, significantly reducing R&D costs. Its advantage in “consistency” gives it a unique competitive edge in scenarios requiring stable character presentation (such as AI-generated short dramas and personalized advertisements). Actively participating in Tencent’s Hunyuan open-source ecosystem for secondary development and innovation is expected to yield first-mover advantages and community support.
- For the General Public: This technological advancement suggests that personalized content creation will become more convenient. In the future, users may more easily transform photos, sounds, or ideas into vivid short videos, greatly enriching personal expression. However, as AI-generated videos become increasingly realistic, the difficulty of distinguishing between real information and AI-generated content increases, making it important to enhance media literacy and critical thinking. From an entertainment perspective, customized animation characters, virtual idol interactions, etc., will bring more immersive, diverse, and interactive experiences.
Recommended Reading https://kr-asia.com/pulses/153192
02 💻 OpenAI Connects to GitHub: ChatGPT Transforms into a “Code Expert” for Deep Codebase Interpretation
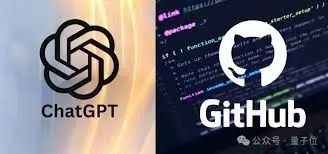
Artificial intelligence leader OpenAI recently announced that the “Deep Research” feature in its popular ChatGPT service has been officially integrated with the GitHub platform. This significant update allows users to associate their GitHub code repositories with ChatGPT, thereby granting ChatGPT the ability to directly read, search, and analyze information such as source code, pull requests, and project documentation within the repositories. Based on its understanding of the codebase, ChatGPT can generate detailed research reports with precise citations according to the user’s specific questions. This move is seen as another key step in OpenAI’s efforts to build ChatGPT into a versatile AI assistant for professional technical users, aiming to significantly enhance developers’ efficiency and experience in understanding, navigating, and operating large and complex code projects. Currently, this feature is available for beta testing for ChatGPT Plus, Pro, and Team version users, with enterprise and education version users expected to gain access soon.
Key Highlights
- Code Insight: ChatGPT can deeply connect to GitHub repositories, analyze code structure, logic, dependencies, and answer users’ specific questions about the codebase.
- Report Generation: Through intelligent retrieval and deep understanding, ChatGPT can automatically generate well-structured and detailed reports, providing code snippets or documentation source citations.
- Efficiency Improvement: Significantly reduces the time developers spend manually browsing, understanding, and searching for information in large or unfamiliar projects, accelerating the onboarding of new team members.
- Permission Compliance: The integration strictly adheres to GitHub user permission settings; ChatGPT only accesses repositories authorized by the user and to which they have access, ensuring code security.
- Strategic Extension: Marks OpenAI’s expansion of AI capabilities from general language understanding to specialized programming assistance and software development support.
Value Insights
- For Practitioners: Developers gain a powerful “super code assistant” that can not only write code but also understand entire codebases. This greatly facilitates learning new open-source projects, refactoring legacy code, or conducting code reviews. Teams can leverage it to quickly generate documentation,梳理 business logic, reduce knowledge loss, and accelerate the onboarding of new hires. AI-assisted programming is evolving from snippet generation to project-level understanding and analysis, indicating a potential profound transformation in software development workflows and productivity paradigms.
- For the General Public: Although primarily aimed at developers, efficient and intelligent development tools can theoretically help developers reduce programming errors and optimize software design, thereby indirectly improving the quality, stability, and user experience of the software products and services we use daily. The “AI understanding complex systems” capability behind this technology has the potential to be transferred to more fields in the future, helping people better understand and operate other complex information systems.
Recommended Reading https://www.techi.com/chatgpt-github-deep-research-integration/
03 💡 Alibaba ZeroSearch Emerges: AI Training Bids Farewell to the “Money-Burning” Mode
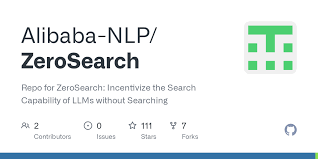
The research team at Alibaba’s DAMO Academy recently unveiled an innovative AI training framework called ZeroSearch. Its core objective is to enable large language models (LLMs) to master efficient information retrieval and integration capabilities by building a simulated search environment for self-training, without directly relying on external commercial search engine APIs. This method utilizes an LLM that already possesses search capabilities as a “simulated search engine” and gradually increases the learning difficulty for the model being trained through a carefully designed “curriculum-based rollout strategy.” The research results are encouraging: LLMs trained with ZeroSearch not only demonstrate performance comparable to or even surpassing models trained with traditional real search engines on multiple benchmarks but, more importantly, can significantly reduce the training costs associated with learning search capabilities by up to 88%. Currently, Alibaba has open-sourced the relevant papers, source code, and models of ZeroSearch on platforms such as arXiv, GitHub, and Hugging Face, aiming to promote the widespread adoption of AI technology.
Key Highlights
- Simulated Search: Utilizes an LLM to simulate search engine behavior, generating relevant and distracting documents for training, avoiding high external API costs and uncontrollable results.
- Curriculum Learning: Employs a “curriculum-based rollout strategy” to provide training documents from simple to complex, gradually enhancing the LLM’s search, filtering, and reasoning abilities.
- Cost Reduction: By eliminating reliance on external search engines, it significantly reduces the training cost for LLMs to learn search capabilities by 88% (e.g., the cost for 500,000 samples drops from approximately $5,000 to only $600).
- Uncompromised Performance: Models trained with ZeroSearch perform no worse than, and even surpass, similar models trained using real search engines on benchmark tests.
- Open Source Sharing: Detailed papers, complete source code, and pre-trained models have been released on mainstream open-source communities and platforms, facilitating utilization by researchers and developers worldwide.
Value Insights
- For Practitioners: ZeroSearch provides a highly cost-effective technical path for developers, especially small and medium-sized enterprises and academic institutions with limited budgets, who wish to equip LLMs with real-time information retrieval and complex question-answering capabilities, helping to break the monopoly of training resources. By building a controllable simulation environment and curriculum learning mechanism, it enhances control over the training process. This “LLM teaches LLM” self-bootstrapping learning paradigm also offers new ideas for solving problems such as data sparsity and high annotation costs in AI training.
- For the General Public: The significant reduction in AI training costs will drive more affordable and powerful AI applications into the market, allowing the general public to enjoy the convenience of AI more widely, such as more intelligent and personalized information acquisition tools. However, since ZeroSearch’s core mechanism does not rely on real-time network information, the AI information it trains may not always be the latest. Users still need to remain cautious and pay attention to the timeliness of information and verify it from multiple sources. More individuals and teams participating in high-performance AI research are expected to accelerate technological iteration and benefit social development.
Recommended Reading https://venturebeat.com/ai/alibabas-zerosearch-lets-ai-learn-to-google-itself-slashing-training-costs-by-88-percent/
04 ⚖️ US AI Chip Export Policy Shifts Direction: Adding New Variables to the Global AI Landscape
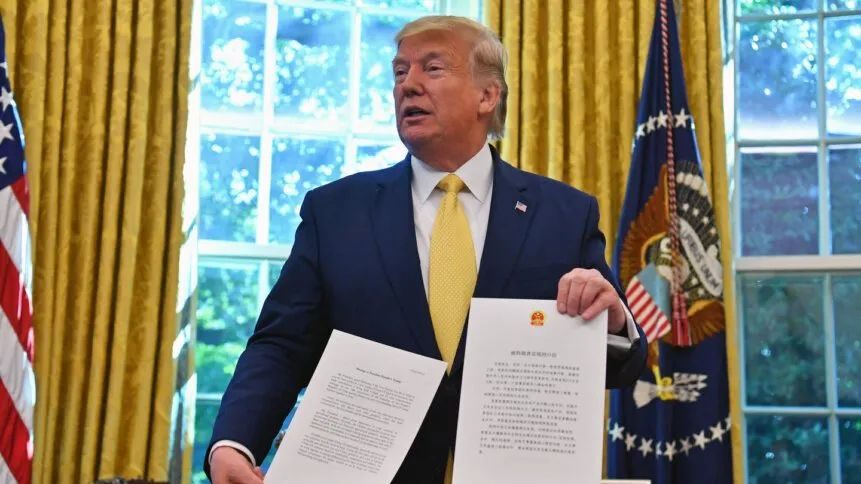
According to recent intensive reports from multiple international media outlets such as Reuters and Bloomberg, the US Trump administration is planning to revoke or significantly modify the “AI Diffusion Rule” formulated and implemented by the previous Biden administration. This rule established a strict three-tiered framework for restricting the export of advanced artificial intelligence (AI) chips to countries worldwide. Sources reveal that the new policy direction may shift towards a simpler, more flexible global licensing system, or manage the export of such high-tech products through intergovernmental bilateral agreements, with the stated goal of “unleashing American innovation potential” and ensuring “absolute American dominance in the AI field.” The signal of this policy adjustment has already elicited positive responses and public welcome from industry giants such as Nvidia. However, this move undoubtedly adds new uncertainties to the already complex global AI chip supply chain and the landscape of international technology competition. Although the specific policy details have not yet been fully announced, it is generally expected that the strict export control measures imposed by the United States on China’s access to cutting-edge AI technology will continue.
Key Highlights
- Policy Shift: The Trump administration plans to abolish or modify the “AI Diffusion Rule” (three-tiered export restrictions) originally set to take effect on May 15, 2025, under the Biden administration.
- New Rule Direction: May shift towards “simpler rules” or rely more on intergovernmental bilateral agreements to manage exports, aiming to promote US innovation and consolidate its AI leadership.
- Industry Reaction: Companies like Nvidia have welcomed this, believing the old rules were complex and bureaucratic, and the new policy holds the promise of historic opportunities. Microsoft and AMD have also previously expressed concerns about the Tier 2 quantity limits in the old rules.
- Unchanged Stance on China: Strict restrictions on China’s access to the most advanced AI chips are expected to continue or even intensify, potentially cracking down harder on transshipment through third countries.
- Review of Biden’s Old Rules: Divided the world into three tiers: Tier 1 (US and 18 partners, essentially no restrictions), Tier 2 (approximately 120 countries, strict quantity limits and authorizations), Tier 3 (China, Russia, Iran, etc., prohibited in principle).
Value Insights
- For Practitioners: Frequent adjustments to the US AI chip export policy bring significant uncertainty to global supply chains and procurement. Chip manufacturers and AI companies heavily reliant on high-end chips need to closely monitor the details, adjust supply chains, market layouts, and R&D directions. If the new policy relaxes restrictions on some former Tier 2 countries, it may bring new market opportunities but also intensify computing power competition. With the overall direction of export controls on China remaining unchanged, relevant companies need to pay close attention to compliance risks.
- For the General Public: This policy adjustment reflects the global technology competition, especially the US-China AI rivalry. The policy outcome may profoundly affect the cost, availability, innovation speed of future technology products, and even national technological strength. Policymakers are seeking a balance between “encouraging innovation” and “maintaining security.” This is a complex and dynamically evolving issue, and its trajectory will shape the future of global technological development.
Recommended Reading https://techhq.com/2025/05/trump-administration-set-to-overhaul-bidens-ai-chip-export-regulations/
Today’s Summary
Looking at the AI industry dynamics of the past 24 hours, a complex picture of accelerating technological innovation and deep intertwining of global strategies emerges. Chinese tech giants are continuously exerting efforts in the field of generative AI. Tencent’s comprehensive open-sourcing of the HunyuanCustom video generation large model not only achieves breakthroughs in the core technology of “subject consistency” but also aims to build a strong developer ecosystem and reshape the application landscape of video creation and even multi-modal AI with its 13 billion parameter scale and thorough open attitude. At the same time, the integration of AI tools and the developer ecosystem is deepening. OpenAI’s connection of its ChatGPT’s “Deep Research” capability with GitHub, the world’s largest code hosting platform, marks a shift from AI assisting in “writing” code to deeply “understanding” code repositories, foreshadowing another significant revolution in software development paradigms aimed at significantly enhancing developers’ productivity and insight.
In terms of the cost and efficiency of AI model training, Alibaba’s proposed ZeroSearch framework offers a new problem-solving approach. Through the “LLM teaches LLM” simulated search and curriculum learning mechanism, ZeroSearch successfully maintains performance comparable to or even surpassing models trained with real search engines while significantly reducing training costs (by up to 88%), and its open-source sharing opens up new paths for the widespread adoption of AI technology. However, the booming development of AI technology is always accompanied by the sensitive interactions of the global supply chain and the profound impact of geopolitics. The proposed adjustment of the US AI chip export policy, shifting from the strict three-tiered control of the Biden administration to potentially more flexible but also clearly targeted (ensuring US dominance and continuing pressure on China) new rules, once again highlights the core position of AI computing power as a national strategic resource. This shift not only affects the nerves of industry giants like Nvidia but also adds more variables to the future direction of the global AI industry chain and the landscape of international technology competition.
Overall, the current AI field is at a critical stage characterized by a surge in technological innovation, accelerated expansion of application scenarios, cost optimization and efficiency improvement becoming key issues, while the global governance framework and industrial policy games are also intensifying. How to strike a balance between encouraging innovation and preventing risks, promoting open cooperation and maintaining one’s own advantages, is a common challenge faced by all participants.